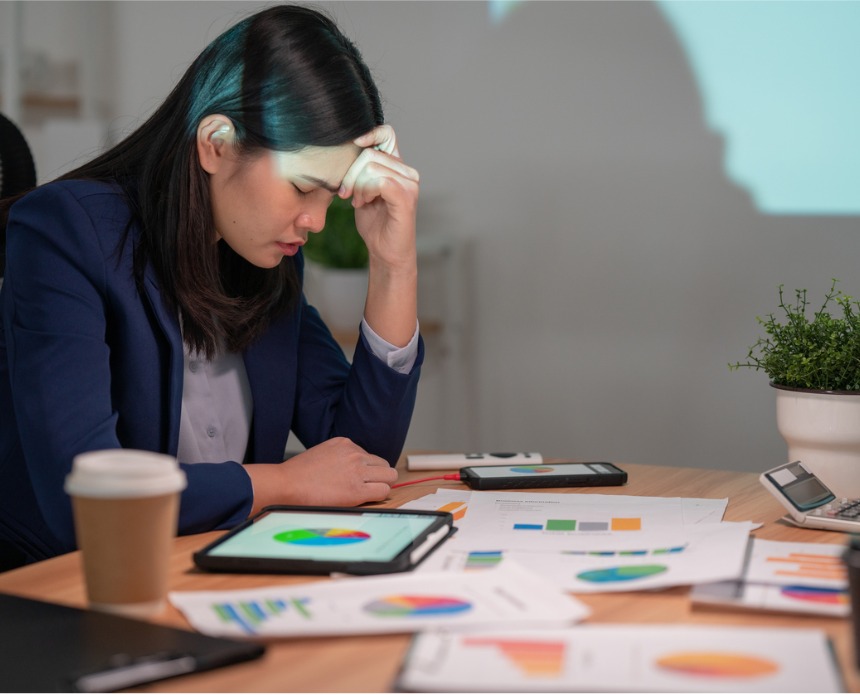
The hidden costs of poor data quality in RevOps
Every RevOps team knows RevOps data quality matters. But few realize just how much it impacts the bottom line. Research from SiriusDecisions puts a precise number on it: for every 100,000 prospect records, organizations with strong data quality generate an additional $390,000 in revenue compared to those with average data quality standards.
Your database: A leaky pipeline
Let’s break down those numbers. In a typical database of 100,000 prospects with average data quality, 25% of records are inaccurate, compared to just 10% in organizations with strong data quality. That means you’re effectively working with 75,000 records instead of 90,000, throwing away 15,000 potential opportunities before you even begin.
The impact cascades through your entire revenue funnel. The research says that organizations with strong data quality see dramatic improvements at every stage:
- 25% higher inquiry-to-MQL conversion rates
- 12.5% higher MQL-to-SQL conversion rates
- 2.6 additional closed deals per 100,000 records
The hidden operational tax across your organization
While revenue leakage is measurable, poor data quality extracts an equally costly “operational tax” that ripples through every department. Marketing teams struggle with campaign targeting and lead scoring, turning what should be precise operations into educated guesses. Attribution becomes nearly impossible, leaving teams unable to calculate true ROI for their efforts.
Sales teams feel the burden most acutely in their daily operations. Rather than focusing on building relationships and closing deals, representatives spend hours manually cleaning and updating records. And every bad record they identify must be replaced by demand generation efforts at approximately $300 per new lead – a hidden cost that quickly adds up. Territory assignments require constant adjustment, and pipeline reviews drag on as teams verify and re-verify questionable data.
The impact on customer success teams is equally severe. Onboarding delays become commonplace due to incomplete customer information, while renewal forecasting turns into guesswork. Valuable expansion opportunities go unidentified because product usage data fails to align with customer records.
Perhaps most concerning is the strain on analytics and operations teams. What should be straightforward reporting tasks turn into time-consuming data cleanup projects that need to be repeated over and over again. System integrations require constant maintenance, and promising automation initiatives fail to launch due to unreliable data foundations.
The challenge grows more complex as organizations expand their technology stacks. With enterprises now averaging 91 technologies in their go-to-market operations, the cost of poor data quality compounds exponentially. Every new tool added to the stack introduces another point of potential data degradation, making each process more brittle and less reliable.
The explosion of GTM data shows no signs of slowing, and will likely accelerate as AI adoption drives even more data creation and collection. The compounding cost of inaction is becoming clearer: modern RevOps teams are realizing that kicking the can down the road is no longer an option. Every day of delay in addressing data quality makes the problem more expensive to fix, while competitors who tackle these challenges gain significant advantages.
What good looks like: the competitive advantage of quality data
Organizations that prioritize data quality are seeing transformative results. When data flows cleanly through systems, lead-to-opportunity conversion times typically drop by 20%. Sales cycles shorten as representatives work with complete, accurate customer information. Marketing teams reclaim countless hours previously spent preparing and cleaning campaign lists, while customer success managers can confidently handle larger portfolios.
The strategic impact is equally powerful. With reliable data, forecasting becomes a tool for confident resource allocation rather than a quarterly exercise in guesswork. Marketing teams can finally trace clear attribution paths, optimizing spend based on real results. Product teams gain true insight into customer behavior, leading to more informed roadmap decisions.
The good news is, a structured approach to data quality can transform this challenge into competitive advantage. Forward-thinking organizations are adopting a comprehensive framework that addresses data quality across three critical dimensions:
- Technical quality: Can you trust the data?
- Operational quality: Can you take action on the data?
- Strategic quality: Can you make strategic decisions with the data?
Time to take action
The math is clear: Poor data quality is costing your organization hundreds of thousands in lost revenue and operational inefficiency. But you don’t have to accept these losses as the cost of doing business. Ready to learn how to manage your GTM data more effectively and create a more scalable technology stack? Download our comprehensive ebook, The Authoritative Guide to RevOps Data Quality, and start turning your data from a liability into a strategic asset.
Recommended Resources
Leave a comment