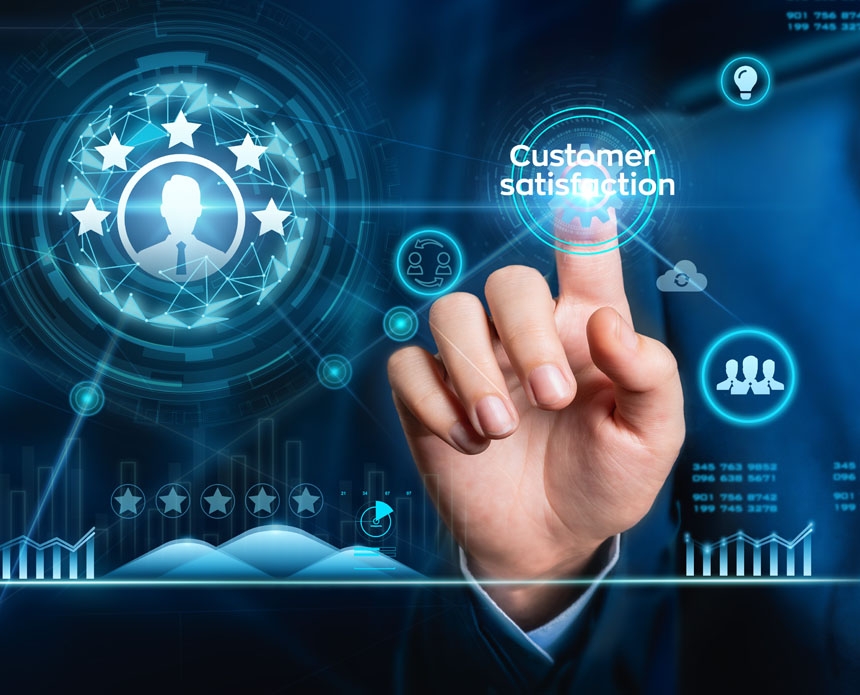
How ICP analysis issues are a data quality problem
In the first post of this blog series, designed to complement The trailblazer’s guide to marketing, sales, and RevOps excellence, we discuss the fundamental role of data governance in ensuring high-quality data. In this follow-up, we shift our focus to how improving your account data quality can resolve many analysis issues and significantly enhance your ability to identify and engage with your ideal customer profile (ICP). Together, this blog series and the ebook provide a comprehensive roadmap to optimizing your RevOps data quality strategy through data management and governance.
How ICP analysis issues are a RevOps data quality problem
So, maybe your analysis isn’t the problem. Maybe it’s your account data quality.
There are clear reasons companies want to identify their ideal customer profile (ICP). If you know you do well with a certain type of company, obviously, you should look at similar companies to try to gain more business! However, determining just which companies belong in your ICP can be a challenge when data isn’t standardized. For example, consider the following set of dirty data:
Company | Country | Employees | Revenue | Industry |
Blue Cat | United States | 5,763 | $502,000,000 | FinServ |
Red Dog | Canada | 500-1000 | 67000000 | Financial Services |
Orange Otter | United Kingdom | less than 500 | less than $50 million | Technology |
Green Hippo | Japan | 10230 | 165,000,000 | High Tech |
Purple Fish | United States | 1285 | $130000000 | Tech |
Yellow Pig | United States | 9,072 | $2 billion | Manufacturing |
The challenge of non-standardized data
If you’re trying to categorize by number of employees, annual revenue, or industry here, you’re out of luck. With the non-standardized values, it looks like you have one in each category for industry and with all the formats in the other fields, it’s hard to know how you’d end up classifying them, if at all. It’s going to take some cleanup and standardization.
Standardizing employee data for better analysis
Let’s start with the employees’ field. First, you need to decide what your format is going to be. What do you want the data to look like when you’re done? For the sake of this exercise, let’s assume you want a number. That means you’ll need to pick a “good enough” value for existing values like “less than 500” and “500-1000”. For these, we can pick something that seems about right (and with the right software in place, this can be automated so you don’t have to clean up each value. You can convert all the values using a step-by-step process that takes care of this for you). You’ll also need to decide if you want to include commas in your formatting for thousands. Let’s say you do, because it’s easier to read numbers that way. So you end up with this (data changes are bolded):
Company | Country | Employees | Revenue | Industry |
Blue Cat | United States | 5,763 | $502,000,000 | FinServ |
Red Dog | Canada | 750 | 67000000 | Financial Services |
Orange Otter | United Kingdom | 300 | less than $50 million | Technology |
Green Hippo | Japan | 10,230 | 165,000,000 | High Tech |
Purple Fish | United States | 1,285 | $130000000 | Tech |
Yellow Pig | United States | 9,072 | $200 million | Manufacturing |
Cleaning up revenue data for clarity
Next, we can clean up the revenue field. We’ll need to make similar assessments. We’ll need to convert words to numbers and determine if we want to include or exclude dollar signs. We could end up with something like this:
Company | Country | Employees | Revenue | Industry |
Blue Cat | United States | 5,763 | $502,000,000 | FinServ |
Red Dog | Canada | 750 | $67,000,000 | Financial Services |
Orange Otter | United Kingdom | 300 | $30,000,000 | Technology |
Green Hippo | Japan | 10,230 | $165,000,000 | High Tech |
Purple Fish | United States | 1,285 | $130,000,000 | Tech |
Yellow Pig | United States | 9,072 | $200,000,000 | Manufacturing |
Standardizing industry values for better categorization
Finally, we can standardize the values for the industry field into a list of options that probably shouldn’t be more than about two dozen, at the maximum; otherwise, it’s hard for humans to make sense of. If there’s a specific industry that your company focuses on, you always have the option to create sub-industry categories for those.
Company | Country | Employees | Revenue | Industry |
Blue Cat | United States | 5,763 | $502,000,000 | Financial Services |
Red Dog | Canada | 750 | $67,000,000 | Financial Services |
Orange Otter | United Kingdom | 300 | $30,000,000 | Technology |
Green Hippo | Japan | 10,230 | $165,000,000 | Technology |
Purple Fish | United States | 1,285 | $130,000,000 | Technology |
Yellow Pig | United States | 9,072 | $200,000,000 | Manufacturing |
Gaining insights through improved RevOps data quality
Now, you can actually categorize your data easily, from country to region (if you have a region grouping for all worldwide countries at the ready), from employees to revenue and industry.
Turns out you have the most business in technology and then in financial services, but manufacturing might not be your sweet spot. You’re doing pretty well with companies between $50,000,000 and $200,000,000, but under $50,000,000 might not be as lucrative. You can filter this data in any number of ways to gain insights about your existing customers and about lookalike companies that might be in your ICP, all because of the improvement in data quality.
Ready to learn more about improving your data quality? Download The GTM guide to data quality to ensure your data is prepped for your RevTech system, or watch our Openprise Master Class on Defining your ICP.
If you’re ready to take the next step, schedule a demo today!
Recommended Resources
Leave a comment