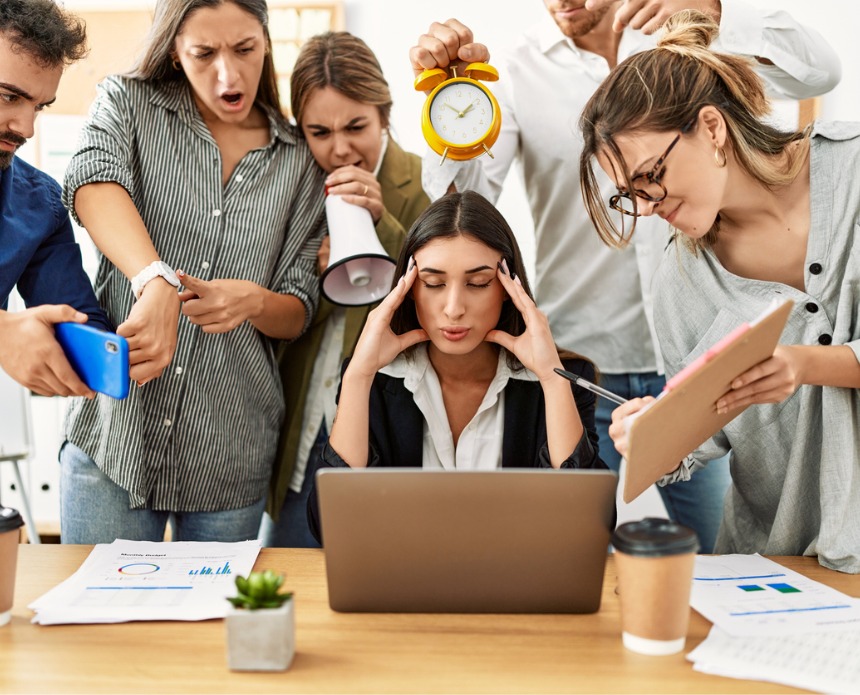
Defining data quality: why your teams can’t agree and what you can do about it
This year, Openprise partnered with RevOps Co-op and MarketingOps to conduct a survey on data quality. This collaborative effort resulted in over 150 responses from operations professionals, giving us new insights into how people define data quality, what holds businesses back from achieving better data quality, and what patterns differentiate teams that achieve good data quality from the rest. Get the full report here.
Have you ever played a game of data “telephone”?
If you work in operations, you’ve played it—whether you realize it or not.
The classic game of telephone starts with one person whispering a phrase to the next. As it passes down the line, details get misheard, misunderstood, or embellished. By the end, the final phrase barely resembles the original.
Sound familiar?
In boardrooms everywhere, this happens with data. Sales reports their lead numbers. Marketing reports a different lead count. Finance has yet another figure. Discrepancies lead to finger-pointing, wasted time, and a growing distrust of data. Instead of aligning around shared insights, leaders start relying on gut instincts—undermining any operations function.
Without stable, reliable data, metrics shift unpredictably. Without a cross-functional approach, leadership teams search for their “truth.” The only way to break this cycle is to establish a clear, shared definition of data quality that aligns with business objectives and drives consistent decision-making.
We previously explored why companies struggle with data quality from both technical and leadership perspectives. Now, we’ll shift gears to the solution: how operations teams can define what truly matters, align data with company objectives, and stop the endless game of data telephone for good.
Not all data is created equal
One of the most cringe-worthy phrases I hear is:
“We want to wait until our data is in great shape before investing in analytics.”
Bad news: if you’re waiting for perfect data, you’ll be waiting forever.
According to the 2025 State of RevOps Survey, 99% of respondents struggle with technical data issues, and 53% say their executive teams believe their data needs improvement. The reality? No company has flawless data—but the most successful ones know how to prioritize what matters most.
The key is focusing on the right data rather than trying to fix everything at once. The most effective companies ensure their most critical data is as accurate and complete as possible while maintaining a realistic outlook on the 80% of data that isn’t essential to decision-making.
How to categorize your data by business impact
Think of data quality in a hierarchy of importance, starting with what directly impacts revenue and customer experience:
- What does my go-to-market team need to deliver a great customer experience?
- Accurate product lists with start and end dates
- A complete record of executed customer contracts
- What does leadership need to make strategic go-to-market decisions?
- Historical wins and losses, along with company and persona profile data
- Market and campaign performance insights
- What does leadership need to guide hiring and product roadmap decisions?
- Reliable opportunity and pipeline data
- Forecasting accuracy
- Customer engagement data (calls, support requests, sales interactions)
- What does my go-to-market team need to execute effectively?
- Firmographic and prospect account data
- Campaign execution details
- Information on common support requests and how to solve them
Leadership may have different priorities, and that’s okay—as long as they understand the trade-offs. If they expect perfection across all datasets while limiting resources, they need to be educated on what’s realistic.
For example:
- Cell phone data will never be perfect. People change numbers, and accuracy will always lag.
- Contract data should always be precise. It’s within your company’s control and directly impacts revenue.
How to define your data priorities
Once you establish a business-first approach to data quality, categorize your data accordingly:
- Mission-critical data: Customer contracts, purchase history, product info, billing data
- High-impact data: Opportunity data, pipeline forecasting, campaign tracking, customer satisfaction and support, core segmentation data (enrichment)
- Customer engagement data: Sales calls, support requests, success interactions
- Marketing data: Ad performance, website analytics, campaign execution details
- Enrichment data: Firmographics, prospect details outside of ideal customer profile factors
- Background data: Anonymous and anonymized website data
By focusing on what truly moves the needle, operations teams can drive alignment, reduce friction, and build confidence in the data that matters most.
Aligning data types with business objectives
The stage and goals of your company will largely determine which data you prioritize first and how you define data quality. Here are examples of both for two types of companies:
Private equity-backed companies: it’s all about profitability & efficiency
Private equity-backed companies often have a short-term exit strategy, aiming for acquisition or merger. These companies focus on demonstrating reasonable growth while obsessing over profitability and strong margins.
To make a company attractive for purchase, minimizing debts and maximizing assets is critical. These are the data categories to prioritize:
- Mission-critical: Billing data, contract accuracy, customer payment history
- High-impact: Profitability metrics, renewal and expansion data, cost per acquisition
- Secondary focus: Sales pipeline health, lead conversion rates, marketing attribution
Here’s a sample data quality definition to align teams:
“Data quality is defined as the accuracy, completeness, and consistency of financial and customer contract data to ensure profitability reporting, operational efficiency, and investor confidence.”
Venture capital-backed companies: it’s all growth and market potential
While venture-backed companies are now more conscious of profitability than they were before 2022, growth remains their primary objective. These companies prioritize data that supports rapid scaling, customer acquisition, and expansion into new markets.
Venture-backed companies should prioritize these data categories:
- Mission-critical: Pipeline and opportunity data, marketing attribution, customer acquisition cost
- High-impact: Lead-to-revenue conversion rates, churn and retention metrics, expansion data
- Secondary focus: Firmographic data for ICP refinement, product usage insights
This example can get teams working from the same data quality definition:
“Data quality is defined as the completeness, timeliness, and reliability of prospect, pipeline, and customer data to drive predictable revenue growth, optimize acquisition strategies, and scale effectively.”
Note that not all prospect and customer data should be prioritized. Focus on what matters from an ideal customer profile standpoint when it comes to enrichment and set a reasonable bar for the rest.
End the data debate
How leaders feel about data quality is just as important as technical data accuracy. Metrics that change as they move through an organization create confusion, inefficiency, and mistrust—just like a game of telephone. The only way to fix it is to ensure every team is working from the same definitions, priorities, and processes.
Make data quality a cross-functional initiative. Align leadership on what matters most. Keep refining your approach as business needs evolve.
When everyone is on the same page, the game stops—and real progress begins.
Take the next step: get the full data quality report
Data quality isn’t just a tech issue—it’s a leadership issue, a business issue, and a revenue issue. The 2025 State of RevOps Survey: Data quality’s impact on GTM execution dives deeper into why companies struggle with data quality and how high-performing teams are solving these challenges.
📥 Download the full report now
Recommended Resources
Leave a comment