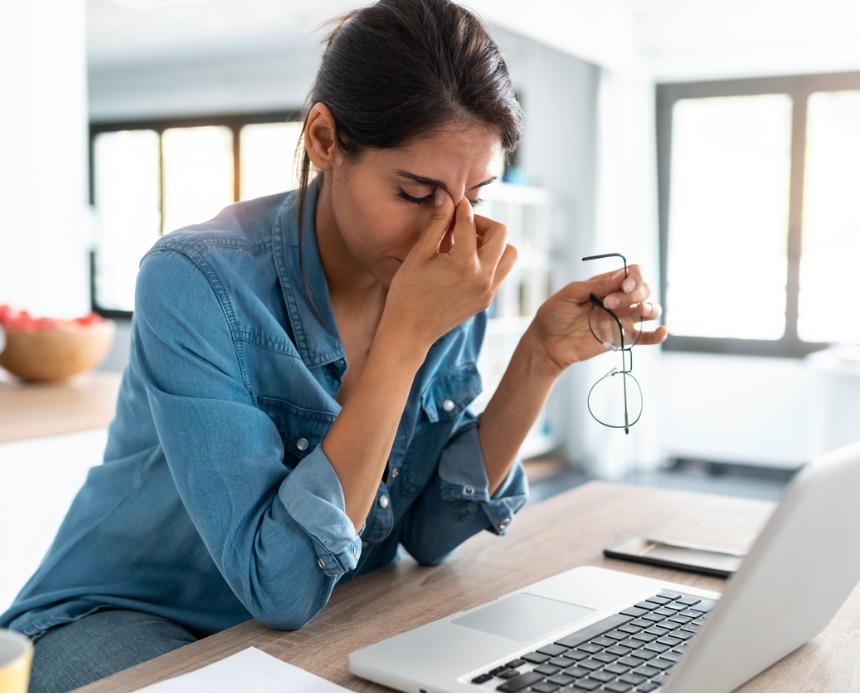
Good enough isn’t good enough: why 89% of RevOps teams struggle with data quality
This year, Openprise partnered with RevOps Co-op and MarketingOps to conduct a survey on data quality. This collaborative effort resulted in over 150 responses from operations professionals, giving us new insights into how people define data quality, what holds businesses back from achieving better data quality, and what patterns differentiate teams that achieve good data quality from the rest. Get the full report here.
Business leaders need reliable information to make smart decisions. But let’s be honest—“data quality” has always been a murky concept.
What does “good enough” data actually look like?
What’s stopping teams from trusting and acting on their data?
And how can we overcome resistance and conflict by eliminating “data quality” as an excuse to dismiss valuable insights?
If this feels a little too relatable (#IYKYK), you’re not alone. We’ve all seen it: leaders defaulting to their gut instincts over data, brushing off insights with a dismissive, “That doesn’t feel right.”
Imagine a world where data is trusted by the executive team and decisions are made with confidence, not skepticism. That’s the future we’re working toward.
That’s why Openprise teamed up with RevOps Co-op and MarketingOps to tackle the big questions:
- What does “data quality” really mean, and how do teams define it?
- What’s holding people back from trusting their data—is it purely a technical issue, or are there deeper challenges?
- What sets high-performing organizations with trusted data apart from those struggling with poor data quality?
We know poor data quality is a problem—it leads to questionable decisions, paralyzes go-to-market teams, and creates friction between departments. But this year’s State of RevOps Survey goes deeper: it reveals why these issues persist, how leadership often (unknowingly) fuels them, and what steps can be taken to break the cycle.
The data is clear: it’s time to stop tolerating bad data and start tackling data quality issues head-on. The insights in this year’s survey provide a roadmap to address these challenges in a meaningful, lasting way.
Why data quality feels impossible (and what’s fueling the struggle)
A staggering 99% of survey respondents admit to struggling with technical data challenges. These include:
- 80% missing or incomplete data
- 75% duplicate records
- 59% non-standardized data
- 52% inconsistent data across systems
- 49% disconnected or siloed data
- 31% outdated or unavailable data
Even with efforts to consolidate SaaS tools in 2022, most businesses still averaged 91 technologies in their RevTech stacks. Managing data across this sprawling ecosystem presents ongoing challenges, such as:
- Juggling multiple enrichment vendors with different data standards
- Merging data from incompatible schemas and identifiers
- Syncing systems without overwriting fresher, more accurate data
- Cleaning up stale or outdated data due to customer churn, company closures, acquisitions, or staff turnover.
And then there’s the wildcard: the very tools designed to manage customer data often make the problem worse.
Take CRMs, for example. Many of the biggest players segment people into two separate, unrelated objects (leads vs. contacts). Add to that their data governance features, which allow administrators to restrict access and visibility, and you’ve got a recipe for chaos—duplicate records, confusion about who’s actually a customer, and an inconsistent customer experience.
One survey respondent summarized it perfectly: “The most frustrating aspect of managing data within our organization is the multitude of systems housing critical information. While some of these systems are integrated, others are not, leading to significant discrepancies.”
Leaders are a big part of the data quality problem
The most compelling insight from this year’s survey is that data quality is as much a leadership issue as it is a technical one.
Hurdles to achieving data quality
A staggering 59% of survey respondents are caught in the crossfire of technical and leadership-related data quality problems. Even more striking, an additional 36% say leadership issues are the only factor holding their organization back. This isn’t just a technical issue—it’s a leadership issue.
Here’s where leadership falls short in organizations with poor data quality:
- 79% don’t have a standard definition of what “data quality” even means
- 55% say adoption of key systems is not enforced by leadership
- 48% report that their leadership team doesn’t understand what is and isn’t possible from a technical perspective
Leaders often fail to prioritize data quality, dismissing it as a low-value initiative or assuming it’s purely a technical issue. This lack of support leads to cascading problems—unaddressed bad data, conflict between teams, and lost revenue opportunities.
As one respondent explained, “The leadership team does not see an issue with data quality and is not putting incentives out for users to adhere to data quality standards. Also, there is no budget for tools or FTEs to deal with this problem.”
The result? Operations teams are left to clean up the mess without the budget, tools, or leadership backing to drive lasting change.
B2b GTM teams: Stop settling for less. Here’s how to fix data quality together
The truth is, fixing data quality isn’t something operations teams can do alone. It requires leadership buy-in, cross-functional collaboration, and a clear roadmap for improvement. Here’s how to get your leaders to lean harder into data quality initiatives:
- Build a compelling business case.
Leadership cares about ROI, so frame the data quality problem in terms of dollars and impact. Highlight how poor data is costing your business—whether it’s in missed revenue opportunities, inefficiencies, or internal conflict—and show the measurable benefits of solving it. - Get leaders to define and own data quality.
Work with leadership to establish a standard definition of data quality for your organization. When leaders co-create the definition, they’re more likely to enforce it across teams. Ownership starts with participation. - Prioritize hygiene initiatives by data type.
Not all data is created equal. Identify which data types have the most significant impact on your revenue engine—whether it’s customer contact details, sales opportunities, or product usage data—and prioritize those for cleanup and maintenance. - Communicate wins to build momentum.
Keep leadership engaged by sharing quick wins and measurable progress. Show how improved data quality is driving better decision-making, reducing inefficiencies, or enhancing the customer experience. Data quality improvements are a journey, but tangible results will keep leaders invested. - Become a data detective (and educator).
Plan to spend time each week identifying the root causes of data “myths” and legitimate issues. Use this as an opportunity to educate your teams on how problems have been addressed, reducing confusion and building trust in the data.
Tackling data quality challenges takes consistent effort and leadership participation—but the payoff is worth it.
Get the full picture
Want to dive deeper into the findings and solutions? Download the 2025 State of RevOps Survey: Data quality’s impact on GTM execution to uncover:
- What’s really holding organizations back from trusted, actionable data.
- How to overcome technical and leadership-related data quality challenges.
- Proven strategies from teams that have successfully solved these problems.
Stop settling for “good enough” and take the first step toward trusted, impactful data. Download the report now and start building the foundation for better decision-making.
Recommended Resources
Leave a comment