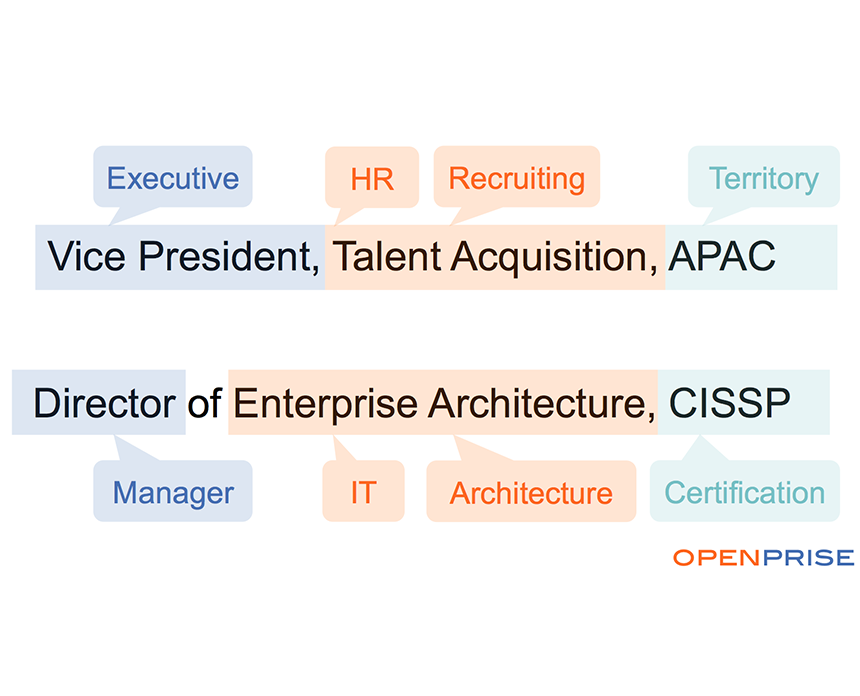
6 quick tips to improve lead database quality
This blog was originally posted on the Marketo Blog, October 9, 2015: https://blog.marketo.com/2015/10/6-quick-ways-to-improve-lead-database-quality.html
Let’s face it—your lead database is dirty and full of holes. It contains duplicate records, incomplete addresses, and limited segmentation. This poor data makes it difficult to create targeted campaigns and engage your users, which ultimately limits what you can achieve with your marketing automation tool. Many marketers think the only way to make their database better is to invest in getting it cleaned by a data vendor. While getting your data enriched and validated by a data vendor is always a good thing, you can make significant and rapid improvements to your data and keep it clean with inexpensive database automation tools.
Here are six tips to improve your lead database by investing just a few hours of your time with data automation technology which can automate the laborious tasks of data cleansing and enrichment:
1. De-duplicate records
Marketers often hesitate to automate de-duplication (the process of removing duplicate leads) and prefer to eyeball duplicates and merge records manually due to fear of eliminating the wrong records. However, if the manual merge process applies a consistent logic–and it should–then that process can be automated. If the merge logic involves many arbitrary “judgment calls”, the result over a large database won’t be any better than automating a consistent logic. The shear effort required to perform manual de-duplication often results in the task never being done at all.
2. Fix bad emails
Emails often fail to send because of typos, like john.doe@company,con. You can revive dead leads by fixing malformed email addresses in an automated way.
Pop quiz!
Question: Is this a valid email address: john.doe@company.com.ot?
Answer: If you have to even put the time into researching whether “.com.ot” is a valid email domain, you need automation. Don’t waste your valuable time on tasks that can be automated.
3. Normalize geographical data
Without complete and normalized geographical data, it is difficult to run regional campaigns, plan field-marketing events, and route leads. For example, trying to build a geographically segmented leads list is difficult when you have to search for every possible variation and typos for often-misspelled state names like Massachusetts, Tennessee, and Mississippi. At a minimum, normalize state/province and country data. Instead of “Massachusetts”, “MA”, “Mass”, and endless other typos, standardize on a single value like “Massachusetts”.
4. Infer missing geographical data
Some data fields have known relationships among them, so having one piece of data allows you to infer missing data. Instead of struggling with incomplete address data, have the system automatically fill in the blanks so that you can leverage these known relationships. For example:
- If ZIP code = 94065, then infer city = Redwood City and state = California
- If country code = 353, then infer country = Ireland
5. Normalize company names
Another common problem with lead database is non-standardized company names. Leads from the same company can have names like “Toyota USA Inc.,” “Toyota Motor Sales USA,” and “Toyota Motors” Normalizing all company names in your leads database is difficult. Instead, focus your efforts on just customer and target account lists. Cleaning up these critical records will provide greater clarity on your pipeline report and marketing metrics overall.
6. Segment using job title data
A well-segmented lead database is the foundation for personalized engagement and targeted campaigns. This includes segmentation by job function, job level, company size, industry, and location. Data services can enrich generic data, but to be effective, segmentation needs to reflect how you sell and whom you sell to. For one marketer, knowing a lead is in IT is good enough, but for another marketer, she needs to know if the lead is in networking, security, or engineering,
One widely available source of segmentation data is job title. By mapping job title keywords, you can create segmentations based on job function and job level. For example:
- “Accounts Payable” indicates job function = Finance
- “CFO” and “Managing Director” indicates job level = Executive
And that’s it. Six quick things you can do now to improve the quality of your leads database without spending a fortune. All you need is a few hours with a database automation tool and reference data like state and country lists and job keyword mappings.
Leave a comment